Neural State Classification for Hybrid Systems
Hybrid Automata (HA) are applied to the design of numerous safety-critical cyber-physical systems, from avionics to medical devices. Formal reachability checking of HAs is thus crucial to derive rigorous proofs of correctness, but current algorithms are computationally very expensive, and therefore, limited to design-time (offline) analysis. This work is motivated by the need to make HA reachability checking more efficient and suitable for online analysis. This is an example of how machine learning can be employed to speed-up complex verification tasks if we are willing to trade strong correctness guarantees (which nevertheless are often only valid at design-time but not at runtime) with approximate verification methods, as long as their error can be formally established.
In the first part of the talk, I will discuss a recently published method called Neural State Classifications (NSC) [1], an approach for approximating reachability checking using deep neural networks (DNNs). In NSC, we train, using examples computed via HA model checkers, DNN-based state classifiers that approximate the result of reachability checking with very high accuracy, classifying an HA state as positive or negative depending on whether or not it satisfies a given bounded reachability specification. DNN-based state classifiers are computationally efficient and suitable for online analysis but may be subject to classification errors, the most important being false negatives (positive states classified as negative). To quantify and mitigate such errors, the approach comprises techniques for certifying, with statistical guarantees, that an NSC classifier meets given accuracy levels; and a newly introduced method for reducing false negatives, based on retraining the network with inputs found by adversarial sampling. In our experiments on six nonlinear HA benchmarks, NSC achieved an accuracy of 99.25% to 99.98%, and a false-negative rate of 0.0033 to 0, further reduced to 0.0015 to 0 after adversarial sampling-based retraining.
In the second part of the talk, I will discuss ongoing research that extends NSC to provide a priori estimates of single-point prediction uncertainty. This is crucial at runtime to flag potential prediction errors without knowing the true reachability value of the inspected state. For this purpose, we apply inductive conformal prediction (iCP), a method that provides statistical guarantees on the predictions of machine learning models. Through iCP, we show how to derive a principled criterion to reject potentially erroneous predictions. This criterion is highly efficient (and thus, applicable at runtime) and effective, managing in our experiments to successfully reject almost all the wrong NSC predictions.
I will conclude the talk with some open problems in NSC.
[1] Phan, D., Paoletti, N., Zhang, T., Grosu, R., Smolka, S. A., & Stoller, S. D. (2018). Neural state classification for hybrid systems. In International Symposium on Automated Technology for Verification and Analysis (pp. 422-440).
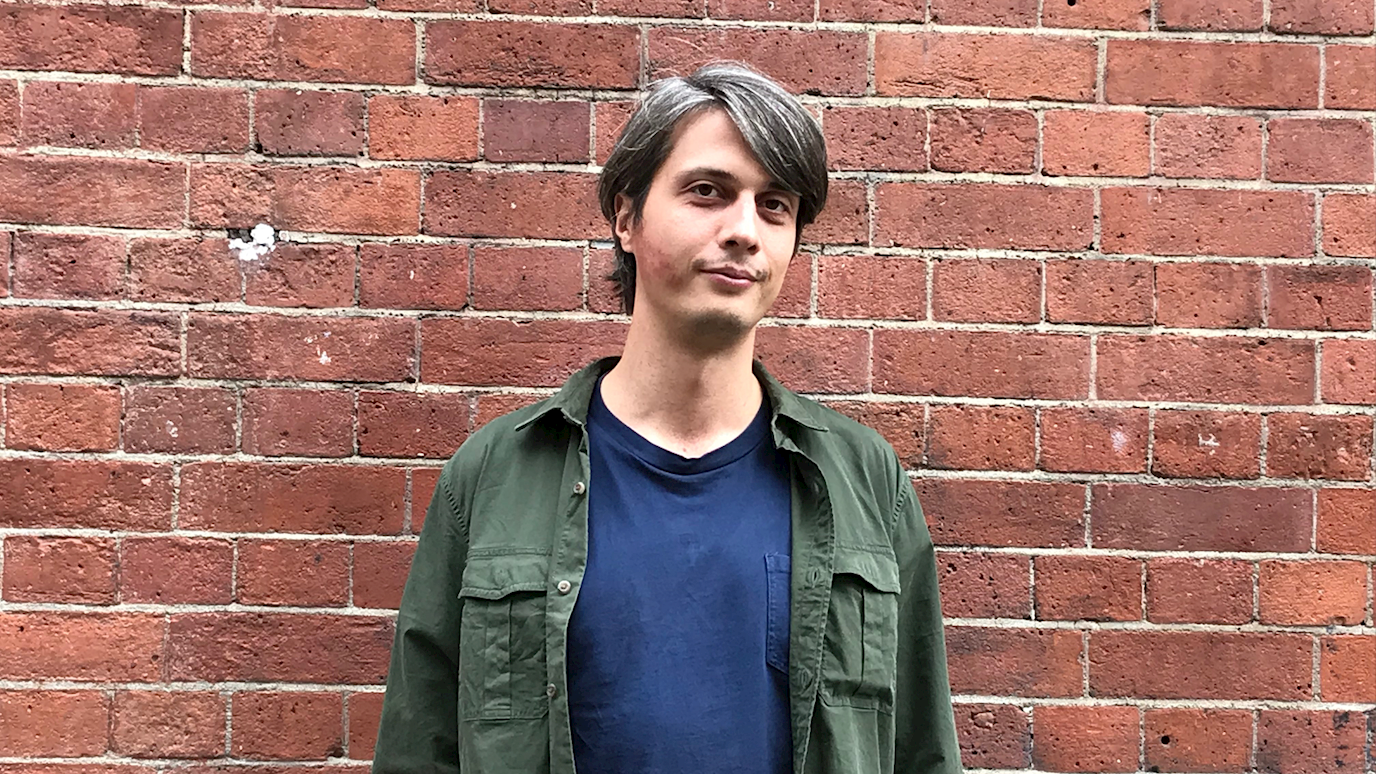
Nicola Paoletti